New paper titled “IPAD: Iterative Pruning with Activation Deviation for Sclera Biometrics” accepted in the Journal of King Saud University – Computer and Information Sciences (SCI IF: 9.00).
Abstract: The sclera has recently been gaining attention as a biometric modality due to its various desirable characteristics. A key step in any type of ocular biometric recognition, including sclera recognition, is the segmentation of the relevant part(s) of the eye. However, the high computational complexity of the (deep) segmentation models used in this task can limit their applicability on resource-constrained devices such as smartphones or head-mounted displays. As these devices are a common desired target for such biometric systems, lightweight solutions for ocular segmentation are critically needed. To address this issue, this paper introduces IPAD (Iterative Pruning with Activation Deviation), a novel method for developing lightweight convolutional networks, that is based on model pruning. IPAD uses a novel filter-activation-based criterion (ADC) to determine low-importance filters and employs an iterative model pruning procedure to derive the final lightweight model. To evaluate the proposed pruning procedure, we conduct extensive experiments with two diverse segmentation models, over four publicly available datasets (SBVPI, SLD, SMD and MOBIUS), in four distinct problem configurations and in comparison to state-of-the-art methods from the literature. The results of the experiments show that the proposed filter-importance criterion outperforms the standard L1 and L2 approaches from the literature. Furthermore, the results also suggest that: 1) the pruned models are able to retain (or even improve on) the performance of the unpruned originals, as long as they are not over-pruned, with RITnet and U-Net at 50% of their original FLOPs reaching up to 4% and 7% higher IoU values than their unpruned versions, respectively, 2) smaller models require more careful pruning, as the pruning process can hurt the model’s generalization capabilities, and 3) the novel criterion most convincingly outperforms the classic approaches when sufficient training data is available, implying that the abundance of data leads to more robust activation-based importance computation.
This is joint work with Matej Vitek, Matic Bizjak, and Peter Peer.
VITEK, Matej, BIZJAK, Matic, PEER, Peter, ŠTRUC, Vitomir. IPAD : Iterative Pruning with Activation Deviation for sclera biometrics. Journal of King Saud University – Computer and Information Sciences, vol. 35, no. 8, pp. 1-1, 2023.
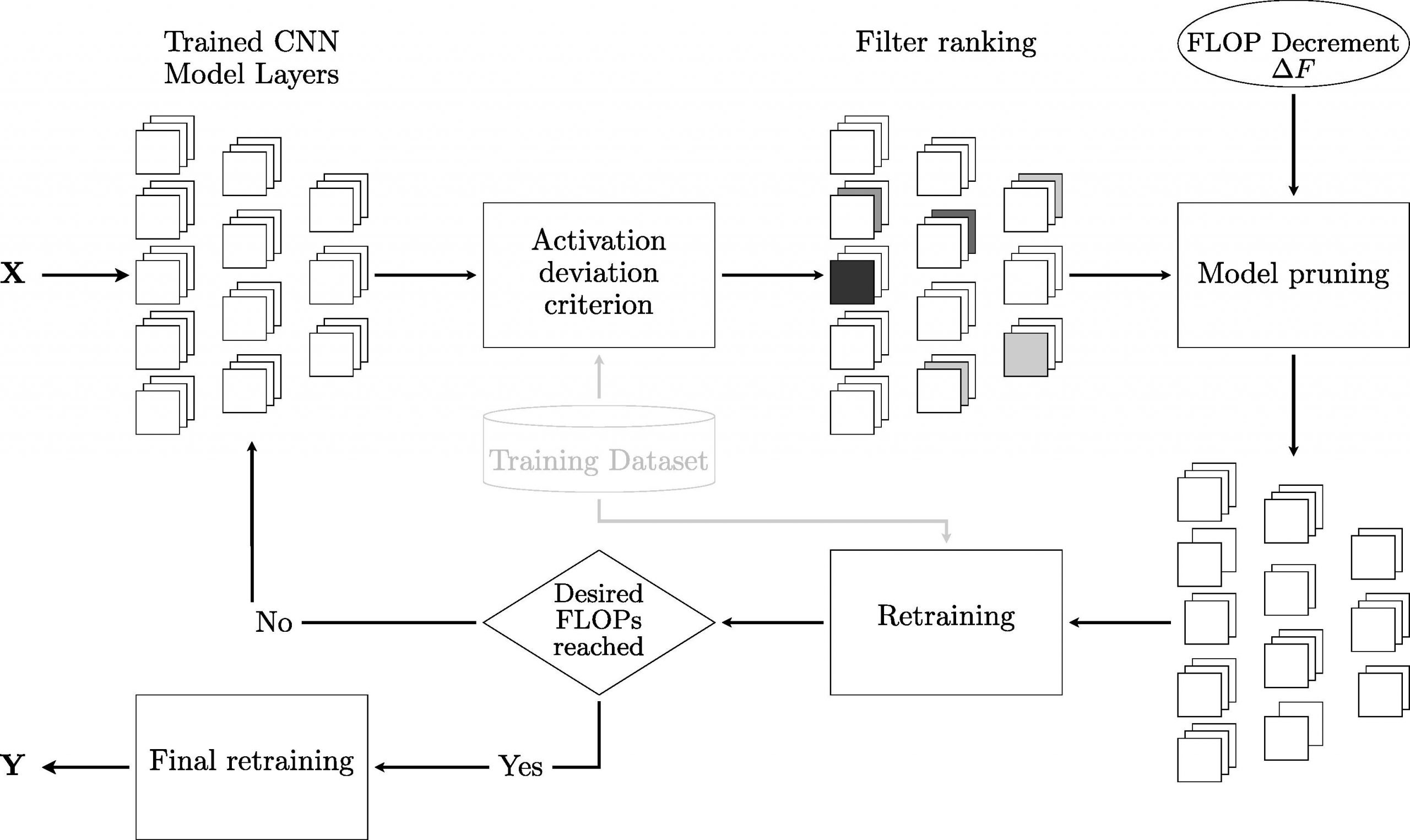