The INFace (Illumination Normalization techniques for robust Face recognition) toolbox is a collection of Matlab functions intended for researchers working in the field of face recognition. The toolbox was produced as a byproduct of the research work presented here and is freely available for download.
The INface toolbox v2.0 includes implementations of the following photometric normalization techniques: the single-scale-retinex algorithm, the multi-scale-retinex algorithm, the single-scale self quotient image, the multi-scale self quotient image, the homomorphic-filtering-based normalization technique, a wavelet-based normalization technique, a wevelet-denoising-based normalization technique, the isotropic diffusion-based normalization technique, the anisotropic-diffusion-based normalization technique, the non-local means-based normalization technique, the adaptive non-local-means-based normalization technique, the DCT-based normalization technique, a normalization technique based on steerable filters, a modified version of the anisotropic diffusion-based normalization technique, the Gradientfaces approach, the Weberfaces approach, the multi-scale Weberfaces approach, the Tan and Triggs normalization technique and the large and small scale features normalization technique.
In addition to the implementations of several state-of-the-art photometric normalization techniques, it also includes a number of histogram manipulation functions, which can be useful for the task of illumination invariant face recognition.
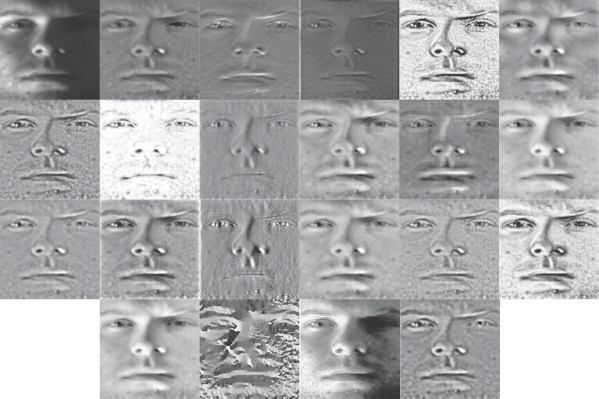
Documentation
Browse through the INFace v2.1 manual HERE.
Download
Download the INFace toolbox from:
Cite
If you use the INFace toolbox for your research work, please cite the following publications:
@incollection{IGI2011,
title = {Photometric normalization techniques for illumination invariance},
author = {Vitomir Štruc and Nikola Pavešić},
editor = {Yu-Jin Zhang},
doi = {10.4018/978-1-61520-991-0.ch015},
year = {2011},
booktitle = {Advances in Face Image Analysis: Techniques and Technologies},
pages = {279-300},
publisher = {IGI-Global},
}
@article{grm2017strengths,
title = {Strengths and weaknesses of deep learning models for face recognition against image degradations},
author = {Klemen Grm and Vitomir Štruc and Anais Artiges and Matthieu Caron and Hazim K. Ekenel},
url = {https://arxiv.org/pdf/1710.01494.pdf},
year = {2017},
date = {2017-01-01},
journal = {IET Biometrics},
volume = {7},
number = {1},
pages = {81--89},
publisher = {IET},
}