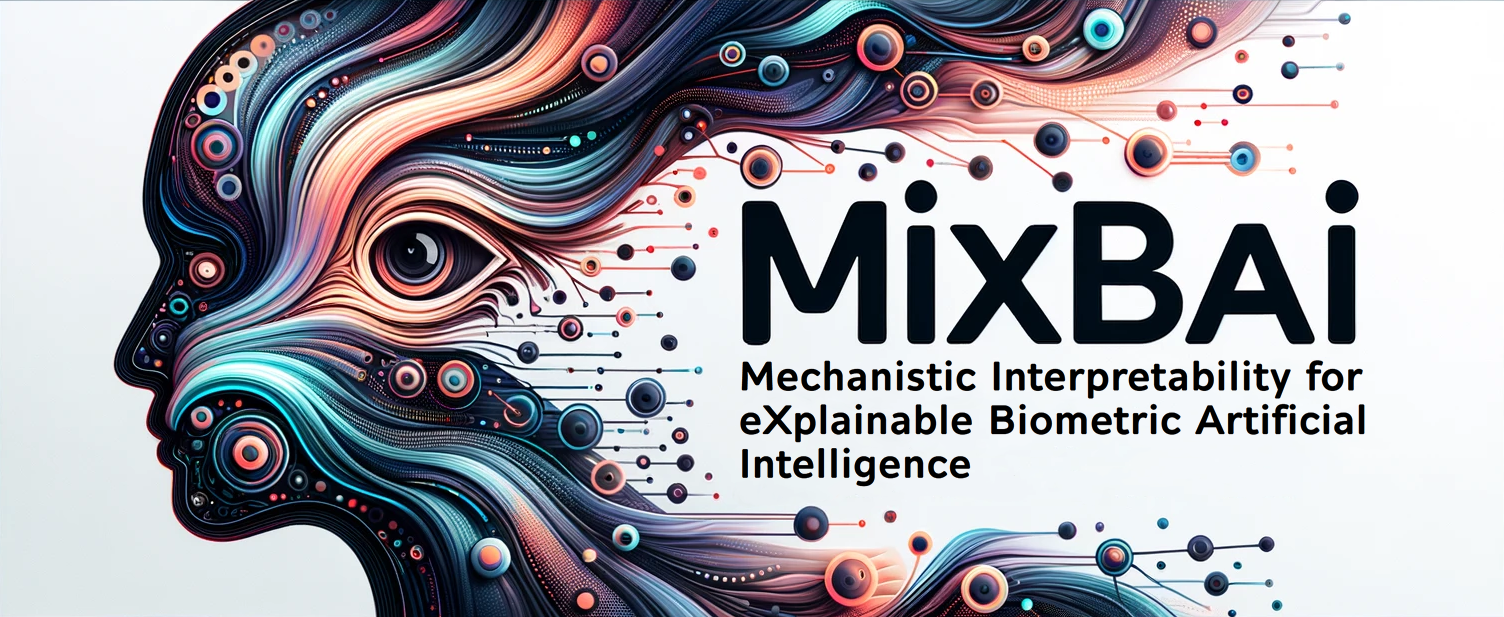
About
The use of biometric systems has grown rapidly in recent years, with applications including, but not limited to, identity verification, access control, surveillance, forensics, medical diagnosis, and personalization. However, the deployment of these systems also raises concerns about privacy, fairness, and accountability. In the European Union, these concerns are particularly acute, given the strong privacy laws and the EU’s commitment to fundamental rights, such as the right to privacy and non- discrimination. Recently, the European commission has proposed a regulatory framework that would establish a risk-based approach to AI, and mandate a right to an explanation for decisions made by AI systems that have profound effects on individuals, e.g., biometric or medical AI systems.
In this context, it is essential that biometric systems are designed in a way that is transparent, fair, and accountable. One key aspect of this is AI interpretability, which refers to the ability to understand and explain the decisions made by artificial intelligence (AI) systems. The development of interpretable, explainable biometric AI systems is necessary to ensure their legal compliance, fair and non-biased decision making, and preserve user trust.
Within the fundamental research project Mechanistic Interpretability for Explainable Biometric Artificial Intelligence (MIXBAI), we are conducting research on AI explainability methods, using the methodology established in the field of mechanistic interpretability. Mechanistic interpretability is a gears-level approach that aims to establish an understanding of the internal workings of otherwise opaque state-of-the-art AI methods such as modern deep neural networks, including convolutional neural networks (CNNs), transformers, and similar methods. The manner in which these methods are developed, i.e., the training algorithms, can result in highly performant decision systems, but ones that often make unpredictable classes of mistakes, making them poorly suited for deployment in high-stakes applications such as biometric systems. Within the scope of the research project, we are developing novel approaches to interpreting the inner workings of state-of-the-art biometric AI systems and explaining their decisions. The developed technology will be able to determine whether advanced biometric AI systems work in a fair and equitable manner that respects user rights, and explain their mechanism of operation in human-readable form.
MIXBAI (ARIS: J2-50069 (A)) is a fundamental research project funded by the Slovenian Research and Innovation Agency (ARIS) in the period 1.10.2023 – 30.9.2026 (1,51 FTE per year).
The principal investigator of MIXBAI is dr. Klemen Grm.
Link to sicris.
Project overview
MIXBAI is structured into 6 work packages:
- WP1: Coordination and management
- WP2: Diving into model interpretation
- WP3: Interpreting discriminative biometric systems
- WP4: Interpreting novel approaches
- WP5: Demos and exploitation
- WP6: Dissemination of key results
The research and development work on these is expected to result in novel interpretability approaches for discriminative models, decision attribution and explanation of biometric AI systems in practical use, and to guide the development of future biometric AI systems in the direction of greater explainability and transparency.
Partners
Work on the project is conducted jointly by
- The Laboratory for Machine Intelligence (LMI), Faculty of Electrical Engineering, University of Ljubljana
- The Computer Vision Laboratory (CVL), Faculty of Computer and Information Science, University of Ljubljana
Participating researchers
- dr. Klemen Grm, PI, LMI
- prof . dr. Vitomir Štruc, senior researcher, LMI
- prof. dr. Peter Peer, PI at CVL
- assoc. prof. dr. Janez Perš, researcher, LMI
- asist. prof. dr. Žiga Emeršič, researcher, CVL
- dr. Janez Križaj, researcher, LMI
- Peter Rot, researcher, LMI
- Žiga Babnik, researcher, LMI
- Marija Ivanovska, researcher, LMI
- Jon Muhovič, researcher, LMI
- Matej Vitek, researcher, CVL
- Blaž Meden, researcher, CVL
- Tim Oblak, researcher, CVL
Publications
Journals
- Žiga Babnik, Peter Peer, Vitomir Štruc, eDifFIQA: Towards Efficient Face Image Quality Assessment based on Denoising Diffusion Probabilistic Models, Transactions on Biometrics, Behavior, and Identity Science (TBIOM), pp. 1-16, 2024 [PDF].
Conferences
- Richard Plesh, Janez Križaj, Keivan Bahmani, Mahesh Banavar, Vitomir Štruc, Stephanie Schuckers: Discovering Interpretable Feature Directions in the Embedding Space of Face Recognition Models. International Joint Conference on Biometrics (IJCB), pp. 1-10, 2024 [PDF]
- Žiga Babnik, Fadi Boutros, Naser Damer, Peter Peer, Vitomir Štruc, AI-KD: Towards Alignment Invariant Face Image Quality Assessment Using Knowledge Distillation, Proceedings of the International Workshop on Biometrics and Forensics (IWBF), pp. 1-6, 2024 [PDF].
Invited Talks
- Vitomir Štruc, Recent Advances in Face Image Processing: From Fashion and Beauty to xAI, Halmstad University, Sweden, April 2024.
Funding Agency
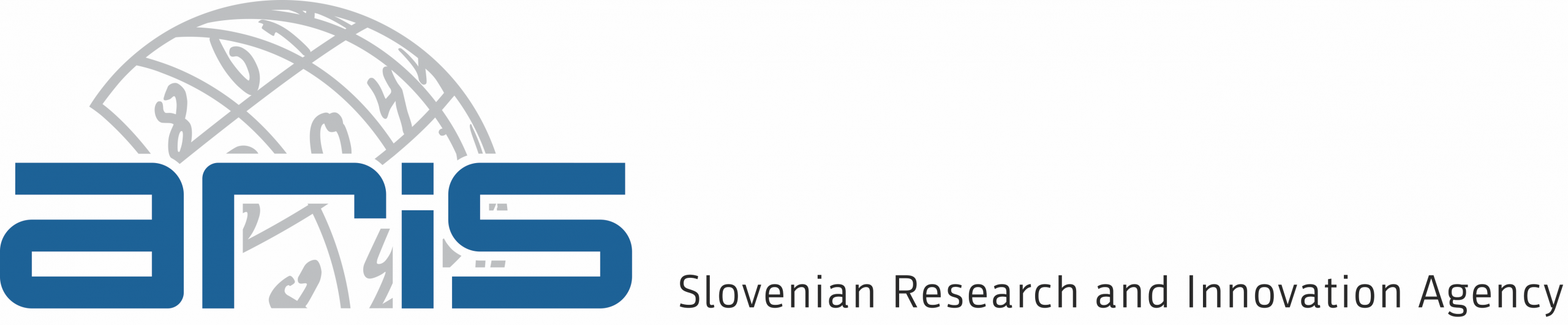